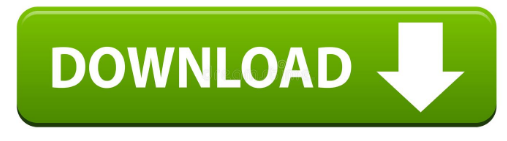
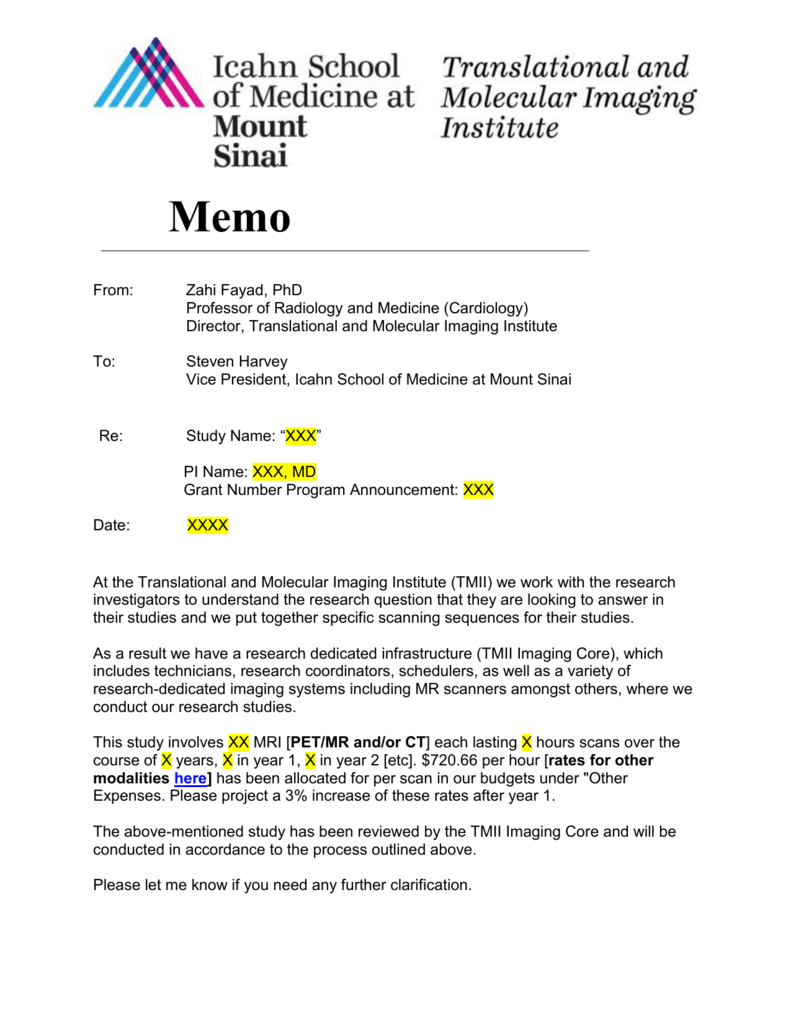
Recently, Tai et al, proposed a Bayesian algorithm which jointly models gene expression from purified cells and that from bulk data through a hierarchical model. To overcome this lack of flexibility, efforts have been made to use semi-reference approaches.

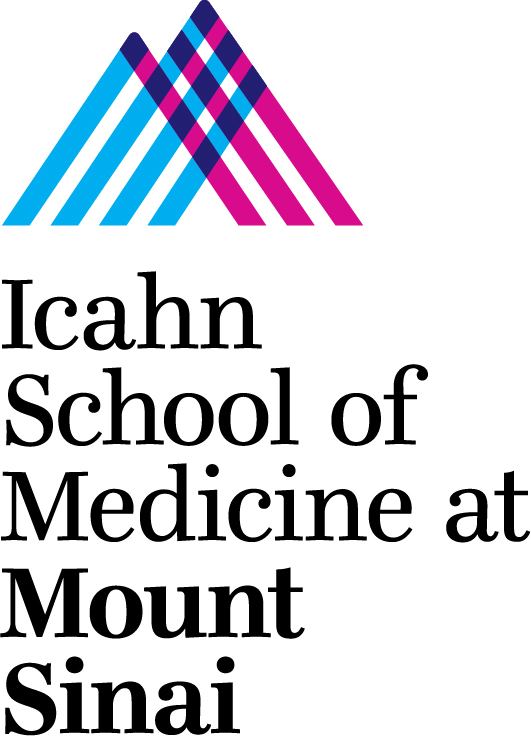
In this context, it is crucial to fully leverage all available data in order to better estimate the immune and stromal composition in the tumor microenvironment. In recent cancer studies, it is becoming common to collect multi-omic data including gene expression and global proteomic data for the same set of biological samples. In addition, this prior information might not be appropriate when performing the deconvolution based on other data types such as proteomic profiles. However, problems might arise when the gene expression of different cell types in certain organs or tumors does not align with those in purified cells.
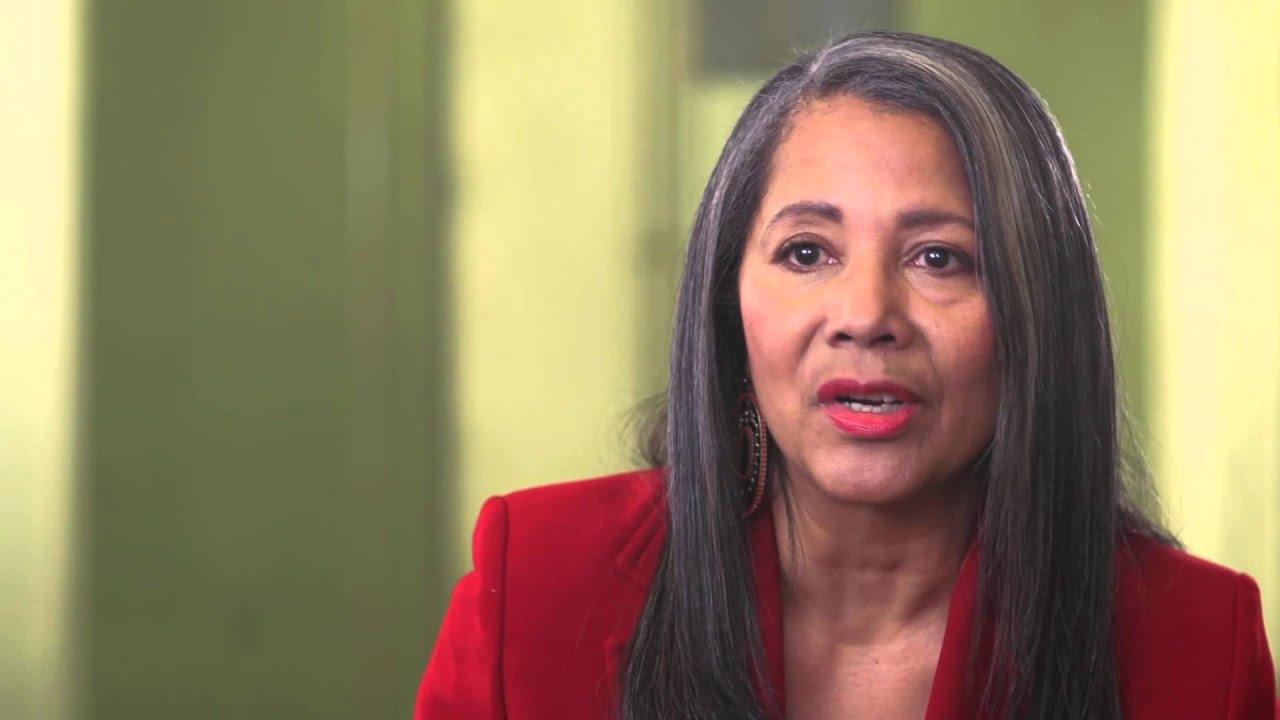
Some algorithms, known as reference-based, require gene expression of purified cells as prior information. In the last decade, different algorithms have been proposed for the estimation of the tumor microenvironment from bulk data. Quantifying the proportion of different cell types in the tumor microenvironment is crucial in order to capture patient heterogeneity and develop better therapeutic targets. Solid tumors are composed of a variety of cell types including immune and stromal cells. Improved performance of BayesDeBulk over state-of-the-art deconvolution algorithms such as Cibersort and xCell is shown on different synthetic and real data examples. This flexible framework enables BayesDeBulk to perform the deconvolution by integrating proteomic and transcriptomic data measured for the same set of samples. Using this prior specification, each component of the mixture model is identifiable and automatically assigned to a particular cell type, overcoming the identifiability issues affecting reference-free methods. Specifically, BayesDebulk imposes a novel Repulsive prior distribution on the mean of cell-type specific markers to ensure the upregulation of cell-type specific markers in a particular component. Results To overcome these limitations, we propose BayesDeBulk, a novel reference-free Bayesian model which flexibly leverages existing information of known cell-type specific markers and performs the simultaneous estimation of cell-type fractions and cell-type gene expression. However, most of these algorithms rely on factor modeling which unfortunately suffers from interpretability issues, as the labeling of different factors into cell types is often problematic. A more flexible alternative is given by reference-free methods which can perform the simultaneous estimation of cell-type fractions and cell-type gene expression from the data. The limitation of these algorithms is that problems might arise when the transcriptomic profiles of different cell types in solid tumors deviate from the reference, leading to poor estimation performance. One class of such algorithms, known as reference-based, requires as input a reference signature matrix containing the gene expression measurements of different cell types. The fraction of different cell types in the tumor microenvironment can be estimated via deconvolution algorithms from bulk transcriptomic profiles. Motivation To improve cancer immunotherapy response, one crucial step is to study the immune/stromal cell composition in the tumor microenvironment.
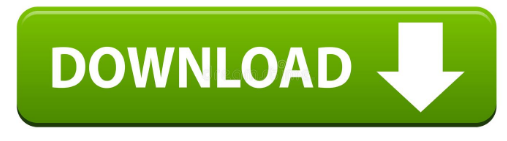